ct_clustprocs Review of cluster procedures.
PRTools and ClusterTools should be in the path
Goto ClusterTools examples for a review of all examples.
Download the m-file from here.
It shows the groundtruth of a 2D example with 10 clusters and compares it with the result of 11 cluster procedures. Evaluation is done by assigning all objects in a cluster to the true class of its prototype.
In CLUSTEX1 the same experiment is coded in a more elaborate way.
Contents
Prepare data and set parameters
randreset % initialise random generator k = 10; % Search for k clusters n = 1000; % Speed up hierarchical and exemplar clustering by reducing % the dataset to at most n points by modeseeking m = 3000; % total number of objects in the dataset x = gendatclust1(m); % generate a 10 cluster problem (3000 objects) a = +x; % create unlabeled data prtime(5) % stop iterations after some seconds
Show the original data with target classes
figure;
lab = getnlab(x);
scatn(lab,a,'Ground truth');
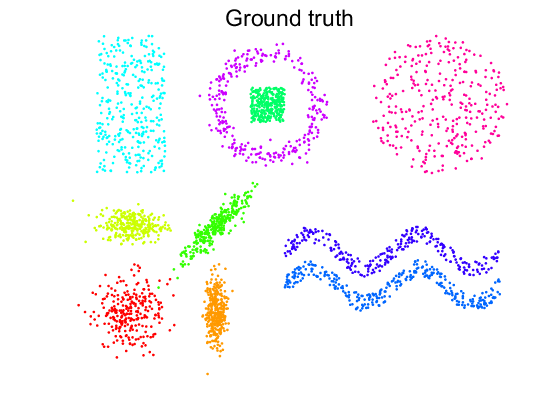
define cluster routines
The cluster routines are defined as PRTools mappings for creating k clusters. For every cluster procedure its scatterplot for 10 clusters is shown. In addition the classification error is printed based on the assignment of all objects in a cluster to the true class of its prototype.
proc = cell(1,11); proc{1} = clustk(k,'KMeans'); % define KMeans proc{2} = clustk(k,'KCenters'); % define KCenters proc{3} = clustk(k,'KMedoids'); % define KMedoids proc{4} = clusth(k,'Single Linkage',n); % define Single Linkage proc{5} = clusth(k,'Average Linkage',n); % define Average Linkage proc{6} = clusth(k,'Central Linkage',n); % define Central Linkage proc{7} = clusth(k,'Complete Linkage',n); % define Complete Linkage proc{8} = cluste(k,n); % define Exemplar proc{9} = clustm(k); % define KNN ModeSeek proc{10} = clusts(k); % define Mean Shift proc{11} = clustf(k); % define FFT figure; set(gcf,'Position',[100 100 1200 800]) subplot(3,4,1) lab = getnlab(x); scatn(lab,a,'Ground truth'); for j=1:11 subplot(3,4,j+1); scatn(ones(size(x,1),1),x,getname(proc{j})); text(8,-2,'... in progress ...','FontSize',15); end % compute results for j=1:11 subplot(3,4,j+1); lab = a*proc{j}; scatn(lab,a,getname(proc{j})); % compute active learning error based on prototypes e = clusteval(lab,x,'actl'); text(10,-2,num2str(e,'%5.3f'),'FontSize',15); drawnow; end
PR_Warning: EXEMPLAR: Examplar clustering updating stopped by PRTIME after 193 iterations
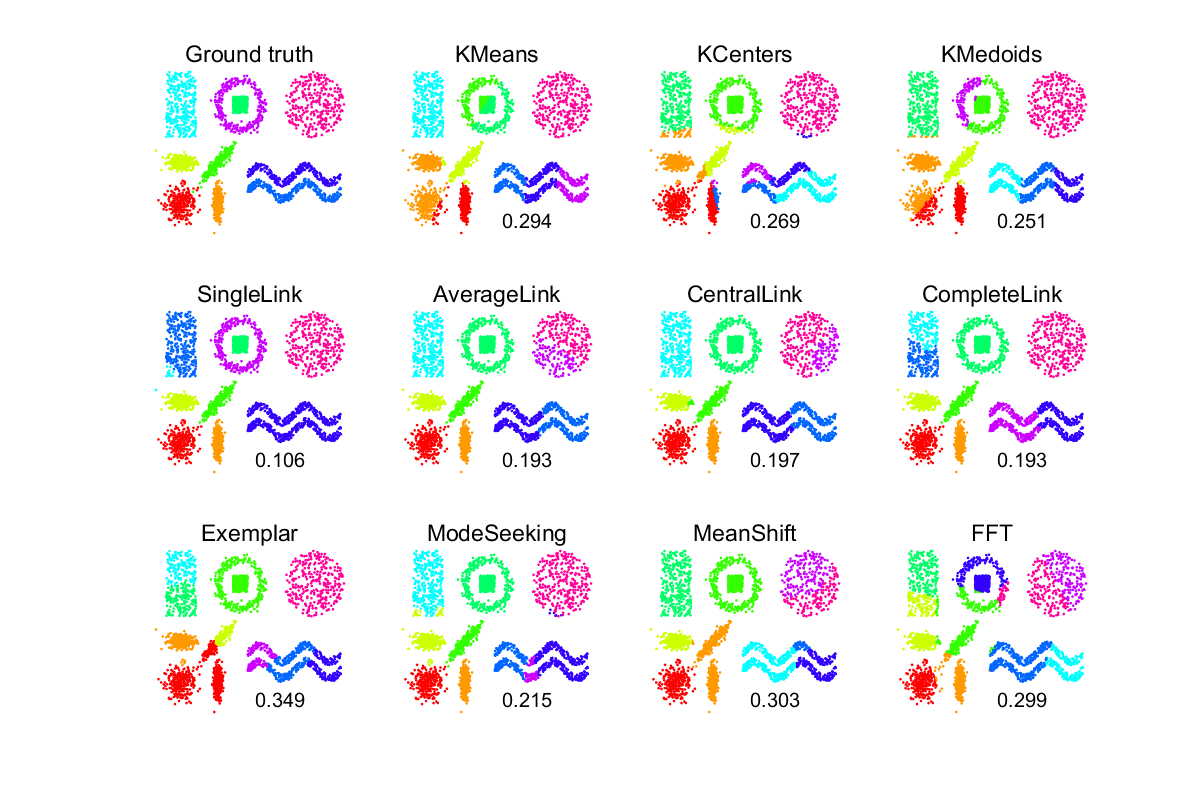
comments
The main purpose of this example is to show how the software can be used. Note that using 100000 (m) instead 3000 objects is also feasible due to the preclustering used in the routines and the default settings of the parameters.